
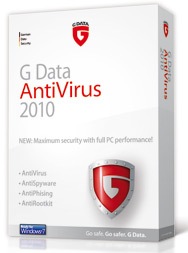
presented a survey of malware detection using data mining techniques. In addition, some surveys have not any systematic classification and article selection for their researches.
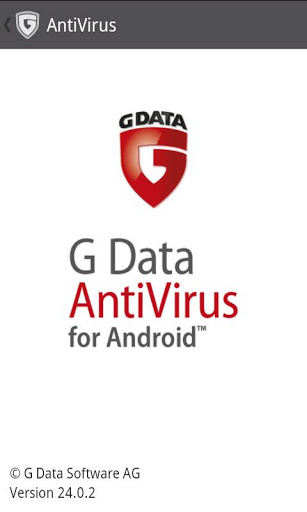
Some papers are published in out of date and did not considered new articles in comparison and analysis. There are some defects in the surveyed research. Of course, some research had discussed the malware detection approaches. In addition, having a new literature review can be influenced on the research studies and explore some technical details in malware detection using data mining techniques. To the best of our knowledge, the most data mining methods have some benefits and weaknesses in malware detection subject. Such a procedure is an important essential with a specific end goal to create effective and powerful identification furthermore characterization techniques malware analysis is partitioned into two primary classifications that include dynamic and static methods. Up to now, malware analysis have the high growing impact in the procedure of deciding the reason and the usefulness the conduct of a given suspicious application. With a large number of malware cases seeming each day, proficiently preparing countless specimens which display comparable conduct, has turned out to be progressively essential.
#G data antivirus 2015 key code#
Typically malware designers don’t compose new code without any preparation, yet redesign the old code with new components or muddling strategies. The most generally utilized malware detection develops a straightforward example coordinating way to deal with identify vindictive code. Malware is a program that makes your framework accomplish something that an assailant needs it to do. The battle between security analyzers and malware scholars is everlasting as innovation grows.
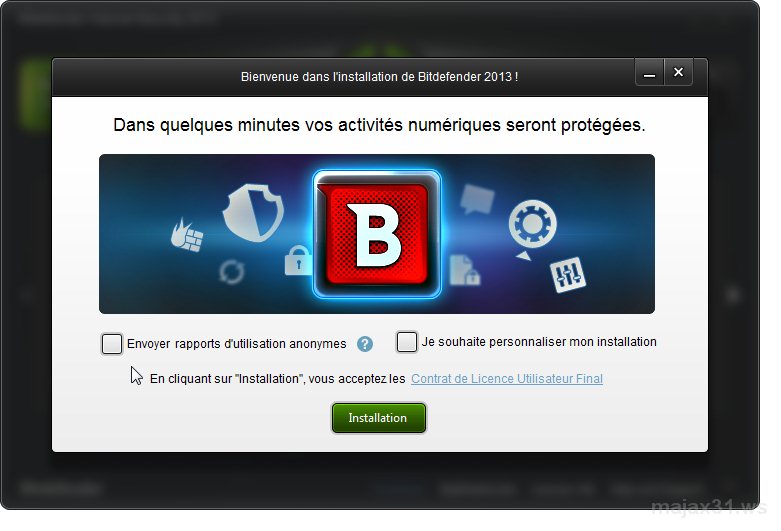
Malware is a standout most thoughtful intimidations for distributed systems and the Internet. These basic examples can separate malware from benevolent code. Machine learning methods can take in hidden examples from a given preparing set which includes both malware and benign examples. In the recent years, the application of malware detection mechanisms utilize through data mining techniques through have increased using machine learning to recognize malicious files. This survey helps researchers to have a general comprehension of the malware detection field and for specialists to do consequent examinations. The advantages and disadvantages of them were discussed in terms of data mining models, their evaluation method and their proficiency. The detection approaches have been compared with each other according to their importance factors. The main contributions of this paper are: (1) providing a summary of the current challenges related to the malware detection approaches in data mining, (2) presenting a systematic and categorized overview of the current approaches to machine learning mechanisms, (3) exploring the structure of the significant methods in the malware detection approach and (4) discussing the important factors of classification malware approaches in the data mining. In addition, it classifies the malware detection approaches in two main categories including signature-based methods and behavior-based detection. This paper presents a systematic and detailed survey of the malware detection mechanisms using data mining techniques. The proposed methodologies are not adequate while evolutionary and complex nature of malware is changing quickly and therefore turn out to be harder to recognize. Data mining techniques have been concentrated for malware detection in the recent decade.
